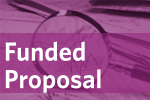
We conduct an audit study to measure the prevalence and impact of AI hiring applications on job applicants. We apply to thousands of jobs, treating half of our application resumes by embedding the job posting within the resume such that machines have access to the job posting text when screening candidates, but human evaluators would not. We can then evaluate if algorithmic resume screening is more likely to select resumes that are a closer match to postings. By also varying applicant race and gender, we can determine if these algorithmic selection algorithms have a differential impact on candidates from underrepresented backgrounds.…Read More