One disadvantage of the gig economy for customers is its fragmentation, making it difficult for consumers and service providers to find suitable matches with each other. In this episode of Mastering Innovation on SiriusXM Channel 132, Business Radio Powered by The Wharton School, Marco Zappacosta, co-founder and CEO of Thumbtack, explains how the company is using digitization and machine learning to standardize service requests and take the guesswork out of hiring the right provider for a job.
When seeking out a service such as a plumbing or house cleaning, customers want an efficient way to hire someone, but they may have a hard time elucidating their specific needs. On the other hand, service providers want to take jobs that best suit their skill set. With competitors like Amazon entering the gig economy, Thumbtack has had to differentiate itself in terms of the unique matchmaking value it can provide. Zappacosta delves into how the company works to effectively bridge the gaps between customer and provider to offer reputable, high-quality services.
Transcript
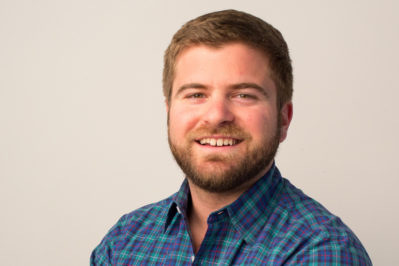
An excerpt of the interview is transcribed below. Listen to more episodes here.
Nicolaj Siggelkow: I just briefly described Thumbtack as a matchmaking service for service providers and their customers. Give us a little bit more detail on what Thumbtack is doing.
Marco Zappacosta: Sure. Thumbtack helps busy homeowners, busy professionals, and busy parents find and hire the local pros they need to get things done. That covers everything you need for your home, from home maintenance to home improvement, as well as things for big events like a wedding or a birthday party. What we do that’s radically different than anybody else is move you beyond just a name and number and instead have a relationship with each of the professionals on the platform, such that, when we show you a pro, it’s because your job matches their interests. We know that they’ve done that work and they’ve done it well. In about 70% of cases, we’re showing you a price up front, an estimate, based on the specific job criteria you have.
The way to think about it is that this has been a historically hyper-fragmented industry and been very hard to assess who’s available, who’s qualified, and how much they’re going to charge, because there was effectively no common platform on which these folks were operating. We are building that [platform]. We are building a place where pros can express their interests, their capabilities, their availability, and their price, such that they don’t have to be queried over the phone or via text message. We can simply query them by API and instantly showcase to the customer the availability that exists around them. This is no different than e-commerce or buying a plane ticket. It’s simply been an industry that has never had this sort of digitization happen to enable this experience.
Siggelkow: In order to make this work, we need information from the customers about what they want, and I think you’ve invested quite a bit in thinking about the right questions to ask. Tell us a little bit more about that.
Zappacosta: Yeah. One thing that I think people don’t appreciate is that there is no concept of an SKU in the service sector. An SKU is a stock keeping unit. It emerged from retailers in the 20th century who needed to manage their inventory and merchandise that inventory. That systematization, categorization, and ontology does not exist in the service world, so we had to create it because it’s through that structure that you enable matchmaking to happen more efficiently. You’re able to bring the two sides together well. If you think about what reduces friction, it’s by having structured answers to structured questions such that you can instantly reveal to your customers the right set of pros, and they don’t have to call each and every name on that list to ask them the same question over and over.
“That systematization, categorization, and ontology does not exist in the service world, so we had to create it.” – Marco Zappacosta
Siggelkow: How did you develop that structure in which to ask the customers the right questions so that the pros can actually know that it is a good fit, or your algorithm can know that it is a good fit?
Zappacosta: The best answer would be slowly.
Siggelkow: Okay.
Zappacosta: It’s very hard because the world is fragmented, and sometimes there are incompatible preferences. One example of that is that housecleaners and carpet cleaners sometimes like to price on a per-square-foot basis. You can appreciate that because that is probably the best predictor of the time that it takes to do that job. The challenge is that most customers don’t know the square footage of their home.
Siggelkow: Correct.
Zappacosta : They know the number of bedrooms and the number of bathrooms. Here is where we face the tension of saying, “How do you systematize this category?” What we ultimately index on is what the consumer knows and is able and willing to provide upfront. Another place where this is intentioned is how you assess a customer’s preference around the amount that they’re willing to spend. How premium or value-conscious are they? The challenge, in this case, is that customers have often never hired this service before.
Zappacosta: When is the last time you hired a water repairman or someone to build you a new fence? Often, you’re hiring these folks and you’ve never hired anybody in that category. So, if we simply ask you the question of how much you are willing to spend, you always low-ball it. You have no idea, so you’d rather say a smaller number than a higher number. It’s this tension of wanting to get the customer to share as much as possible but also recognizing that they don’t have all of the information. We have to meet them halfway and help them get there over time.
Siggelkow: Yeah. That’s one half of the equation, getting the information from the customer so we can have an idea about how a good profile would look on the other side. How do you get the data on the other side? Now we need to understand who these pros or contractors are, what they can provide, and what they want. Are they selecting the match, or is your algorithm selecting it?
Zappacosta: The way that it works is that they tell us the criteria and the things that are binary: things they will or will not do. You could imagine, if you’re a moving company, you have a limit to the number of miles that you’re willing to move a customer or the size of the job. That is a hard constraint and those they express explicitly. But then there are the soft preferences. This is where, through machine learning and the data we see, we’re able to tune the system to not simply be a reflection of their stated preferences but of their revealed preferences. If we see that they are engaging with a certain type of job more or less often, that will factor back in. If we see that customers are engaging with them more or less for certain types of job, that will also factor in. The pro tells us what they’re willing to do, and then the system ideally helps make for the best match, knowing what the customer is looking for and knowing what the pro is best suited to do.
“Through machine learning and the data we see, we’re able to tune the system to not simply be a reflection of their stated preferences, but of their revealed preferences.” – Marco Zappacosta
Siggelkow: One key problem in this market is reputation, right? I, as a customer, have no idea. As you just said, I’m not in the market for plumbing services a lot or for building whatever it is that I need to be built in my house. How do you help the customer overcome this big pain point, the reputation of the people on the other side?
Zappacosta: Trust is one of the key factors when customers make these hiring decisions. The way we think about it is that there are two core pieces to giving you peace of mind as a customer. The first comes down to the pro being who they say they are and being a trustworthy human being. This is something that we take on as a platform and ensure that every pro who comes through meets our standards. We are confident that we know who this pro is, we’ve ascertained their identity, and we’ve checked that identity in enough ways to be confident that they are an upstanding human being. But that is only one piece of it. Simply being safe is not enough, you also want someone who delivers quality service.
This second part is where the community ratings come in. Ultimately, that is a reflection of the quality of service that this pro is delivering to our community of customers. The one wrinkle there is we let pros gather a maximum of 10 testimonials to help them get started. Otherwise, there would be this challenge for a new pro who may be great but not have any reputation to jump-start their engagement. And so we let them solicit 10 testimonials from past clients and, after that, 100% of the reviews come from Thumbtack customers. The thing that I think adds a lot of robustness to these reviews is that they’re tied to a specific job request. So we know that you, as a moving company, have done 12 other jobs exactly like this and have gotten five stars for all of these jobs. We also know the ZIP code of where your previous clients were and where the current one is, and ZIP code is a good proxy for preferences. And so we’re able to infer a lot from that.
At the core of it, it’s investing heavily from a trust and safety standpoint to ensure that all actors on the platform are upstanding and meet our guarantees, then getting enough community data so that we can make the best matches possible and be very transparent. Our reviews have pictures of the projects, and they have prices, unlike a directory. We are building a very unique and very rich review data set, which is critical to giving customers the confidence to hire.
About Our Guest
Marco Zappacosta is the cofounder and CEO of Thumbtack, a service that connects customers who need something done with the right professionals. Recognized by Forbes as one of 2015’s 30 under 30, Marco has been featured in stories in the New York Times, Fortune, the Wall Street Journal, and CNBC, and Thumbtack was recently acknowledged as one of GlassDoor’s best places to work. Marco started Thumbtack after graduating from Columbia University, where he majored in political science (after failing the last class of his neuroscience and behavior major). He and his wife live in San Francisco.
Mastering Innovation is live on Thursdays at 4:00 p.m. ET. Listen to more episodes here.
Do they ever match people in regards to romantic relationships?
Deliberately? Not that we know of. Unintentionally? Maybe sometimes!