When people consider the future of artificial intelligence, one frequent point of discussion is the law of unintended consequences. Science fiction has vividly illustrated the fear of machines becoming sentient and turning against their creators, but even in reality we’ve already seen unanticipated problems emerge — in HR machine learning applications that incorporate and amplify human biases, for instance.
On this episode of Mastering Innovation on SiriusXM Channel 132, Business Radio Powered by The Wharton School, we spoke with John Roese, President and CTO of Dell EMC. Roese explained that advances in AI will depend on earning human users’ trust in machine learning. For example, applications that involve human interaction might one day incorporate sensors and biofeedback to essentially build empathy for the operator into the system. Until then, Roese said, advances will remain mostly incremental, though still meaningful.
An excerpt of the interview is transcribed below. Listen to more episodes here.
Transcript
Harbir Singh: There’s a big issue around machine learning, which is really based on decisions people make, right? The algorithms learn from decisions and embed them into their programming. How do you make sure that there’s a level of focus in that it doesn’t grow beyond its role and become larger and less manageable, even as it tries to make other technologies manageable?
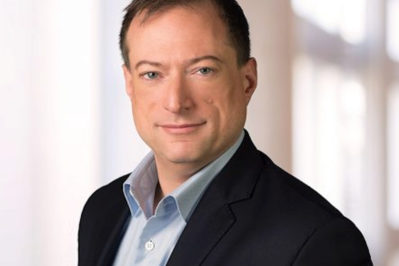
John Roese: That’s the long-term dilemma with machine intelligence and this idea of shifting cognitive tasks onto machines. Remember, for the last 200 years we’ve divided the labor of the world as follows: thinking tasks are done by people; mechanical tasks should be done by machines. In the last five years, we’ve entered a new era where we’re actually dividing up the thinking tasks. The good news today is that we’re nowhere near artificial general intelligence where things get a little more interesting. But what we do have to determine is in applying artificial intelligence to a problem, we need to bound that problem and understand what we’re getting, because the unintended consequences of losing visibility into a particular area can be problematic. Most of the AI projects in the world, ones that are actually meaningful and do something that has an outcome (as opposed to simply academic and forward-looking, which are also very important) tend to be things that achieve a 5% or 10% improvement on a particular decision-making task.
They aren’t orders of magnitude better than a human being, but they are better enough that it’s worth using that tool. But their task is very well defined. They do image recognition slightly better, or they do caching slightly better. Now, those will continue to evolve, but because they’re bounded to a domain — for instance, my AI logic in my storage systems to do caching — it’s not going to become the Terminator. It’s not going to do something completely out of the box, because the data it’s exposed to and where it sits in the business process is very well defined. We’re probably going to stay in that phase for quite some time.
Even within that, there are problems. For instance, what we’ve discovered is that if you apply machine intelligence to make decisions in something like HR hiring — this is commonly discussed these days — if you lose visibility and understanding of the datasets and you don’t really understand how the logic is happening, the incorporation of bias into the data streams could result in bias in the output. This basically results in hiring the wrong people and creating their own demographic, and that’s catastrophic.
Inside of Dell, we’d had this discussion. What we concluded was that we’re going to tread very carefully. In the meantime, we’re going to do a whole bunch of machine intelligence projects on things that are much better defined and that have a far lower risk profile. Because to be candid, every decision-making process in our company, from supply chain, to HR, to development, to how our products work, could be improved through the tactical and strategic incorporation of machine intelligence. So today it’s really, I hate to say it this way, go after the low-hanging fruit. Find the ones that you can quantify and control. That’ll keep you busy for a few years.
As the industry evolves to develop better approaches to bias detection or mediation, it will be able to create better security and bounding and policy models around AI, and even to create a set of ethics that the industry can agree on around the use of very advanced AI. Those issues will work themselves out. By the time mainstream industry needs to do that, I’m optimistically confident that we will have better tools and better environments. But in the meantime, we can be very busy improving our businesses and actually invoking the next wave of productivity into the economy.
About Our Guest
John Roese is the President and Chief Technology Officer of Dell EMC. In this role, John is responsible for ensuring the company anticipates customer needs and provides the essential infrastructure for organizations to build their digital future, transform IT and protect their most important asset, information. John and his organization provide the thought leadership and future-looking technology strategy needed to foster innovation and boost Dell EMC’s infrastructure technology and R&D capabilities, working collaboratively with the Dell Technologies family of businesses.
John is a published author and holds more than 20 pending and granted patents in areas such as policy-based networking, location-based services, and security. He currently serves as the Chairman of the Board of Cloud Foundry Foundation, the leading open source platform for cloud native applications and was recently named one of The World’s First Top 50 Edge Computing Influencers by Data Economy Magazine.