To discover new music, there are Pandora and Spotify, and to discover new movies, there is Netflix. How would one go about discovering new art? In this episode of Mastering Innovation on Sirius XM Channel 132, Business Radio Powered by The Wharton School, guest Carter Cleveland, Founder and CEO of Artsy, discussed how Artsy digitizes the process of finding and acquiring art.
When selling a work, artists often face inefficiencies in the forms of loss of time and value. According to Cleveland, the average artists gives up 30-40% of the total price and can wait up to six months to sell a piece. To help today’s art consumer get access to the full market, Artsy aggregates supply and demand for potential sellers and buyers. Inspired by Pandora’s Music Genome Project, Artsy has also introduced the Art Genome Project, categorizing pieces by their historical movements and leveraging artificial intelligence capabilities, such as similarity algorithms and collaborative filtering, to make recommendations. Cleveland explained that on-boarding suppliers like galleries and auction houses was initially difficult, but Artsy pursued a partnership strategy rather than a disruptive one, which proved to be successful.
An excerpt of the interview is transcribed below. Listen to more episodes here.
Transcript
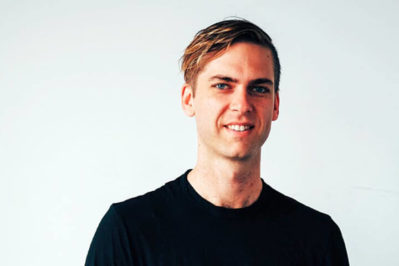
Carter Cleveland: Most people see art as something that’s intimidating and scary. It’s hard to understand. As I was looking online for better resources to learn about and discover art, I realized that while we had sites like Pandora or Spotify that aggregate all the music and sites like Netflix that aggregate all the film, there was no single website that aggregates all the world’s art in one place to make it easy to learn about, but also discover new artworks and new artists.
A lot of my interest was in the discovery part because I was majoring in computer science engineering. I was particularly fascinated by artificial intelligence. In fact, I was originally [majoring in] physics, and I switched out of physics into computer science – literally because I read the course description for AI. I had been very closely following how Pandora had done music recommendations and how Netflix had done it for film. I realized that no one had systematically gone after the category of art in order to understand it and make intelligent recommendations around it. That was a lot of what attracted me to it. It was the academic interest in the algorithms around recommending arts, hence, the relationship to Pandora.
Since then, we created the Art Genome Project. We modeled that after Pandora’s Music Genome Project. We have a team of people that categorize all our artists and artworks based on which art historical movements are relevant to them, and that’s the basis for our recommendation.
Nicolaj Siggelkow: How is that classification evolving over time? I know you started out by creating scales and creating dimensions. How has that project evolved?
Cleveland: That’s a great question. As you might imagine, you start out with the basics of art history. Say someone is looking at a work by Andy Warhol. If you are an art adviser, you might want to also recommend a work by Tom Wesselmann or maybe Roy Lichtenstein – essentially, other artists related to the pop art movement. What we did is, we took those main categories – major art historical movements like pop art or abstract art, expressionism or cubism, or different contemporary movement – and we started categorizing artists, giving scores to both artists and individual artworks across all these different art historical movements. It uses a genetic similarity algorithm, essentially: how similar are these artworks or artists based on their genome? You know what to recommend to people based on what they’ve already looked at.
“There was no single website that aggregates all the world’s art in one place to make it easy to learn about.” – Carter Cleveland
Over time, one of the main things is, as you get more and more art, you have to expand the art genome. Initially, we had a handful of galleries. Now, we have thousands of galleries and hundreds of thousands of artworks. If you’re an art historian, you might start off in one department, but as you move into different geographic regions or periods of time, you have to understand or develop new art historical movements in order to understand the contextualized different regions and periods. So, the genome is constantly expanding.
We call each movement or category a gene. We might notice, “Hey, these three genes are very similar. Let’s actually combine them into a single gene.” We’ve also applied some machine learning to try and better understand how you can predict certain genes from the visual quality of work. For instance, you can imagine a gene for bright colors or for colors in general. That’s very easy to extract from the visual data of the artwork. You can imagine that a gene like geometric, which describes a very specific visual quality of a work, is another type of gene that can potentially be categorized using artificial intelligence versus human. It’s always something that we’re trying to get better at expand our knowledge in.
Sigglekow: That was actually my next question. It sounded like you might have started out like Yahoo, by having people classify things into categories. Then, you used the algorithms to make predictions of, “If you like A, you may like B,” and find another tree of knowledge of a genome. How much have you turned to AI to make the classification in the first place?
Cleveland: We’ve expanded it in a few ways. I would say that the vast majority of the categorization we do is still done by human. But as I said, the more visual the characteristic is, the easier it is for machine. The other thing we’ve done is we’ve looked into using collaborative filtering at the artist level. Collaborative filtering is the algorithm that Amazon uses to say, “Other people who like this also like this.” Collaborative filtering is the best-in-class algorithm for e-commerce where you’re selling skewed items or mass-produced items. The challenge of applying that to unique artworks, or to unique anything, is that once people have bought that one other thing, it’s gone. If you send more people to it, you’re just sending them to a sold artwork.
“With art, you have to be able to make recommendations for things that have not yet have user interest or user purchasing behavior on them because once they buy it, it’s gone.” – Carter Cleveland
With art, you have to be able to make recommendations for things that have not yet have user interest or user purchasing behavior on them because once they buy it, it’s gone. Hence, the need for genome similarity. However, you can apply collaborative filtering at the artist level. You can say, “You like Andy Warhol. We’ve noticed that people who like Andy Warhol also tend to like Tom Wesselmann or Roy Lichtenstein.” Those are other ways that we’ve expanded how we do recommendations there.
About Our Guest
Carter Cleveland is the founder & CEO of Artsy, the leading global platform for collecting and discovering art. Cleveland founded Artsy in his dorm room when he was a computer science student at Princeton. Cleveland’s concept — a free website for art powered by The Art Genome Project, a classification system mapping characteristics between artists and artworks as a tool for discovery and learning — earned him second place in a school competition and funding to assemble Artsy’s founding team of art specialists and engineers. Since launching in 2012, Artsy has grown into the world’s largest art marketplace, partnering with more than 2,500 galleries, 700 museums, 60 international art fairs, and top auction houses including Phillips and Christie’s. Artsy has over 200 employees across three continents and has raised over $100M in funding backed by influential figures in art, technology, and business.
Mastering Innovation is live on Thursdays at 4:00 p.m. ET. Listen to more episodes here.