Understanding the Network of Causality to Develop a Smarter Forecasting Strategy
The following Q&A is a guest post written with Wendy Ham, whose research was funded by the Mack Institute in 2014.
The overarching question in my research is: What are some factors that influence forecast accuracy? Specifically, I’m looking at forecasts of binary events, that is, events that can be framed as having only two possible outcomes — either it occurs or it does not. Since we are in the election cycle, one example of such an event might be: “Donald Trump gets the presidential nomination.”
There are essentially two main categories of factors that influence forecast accuracy: The first are the characteristics of the event that a forecaster tries to predict, and the second are the characteristics of the forecasting method. In the example above, the event is, again, “Donald Trump gets the nomination,” and the method could be anything from statistical analysis of tweets to pundit evaluation of late-night talk show appearances.
With regards to the first category, my questions are: When given a forecasting challenge, can we figure out in advance how difficult the task is going to be? And if so, how? In a sense, my research in the first category can be paraphrased as an attempt to understand how to “predict predictability.”
With regards to the second category, my questions are: What are some common sources of imperfections in forecasting methods, and how do they influence accuracy differently?
Ultimately, the practical question is: Can we make a reliable cheat sheet that tells us what sort of forecasting method is the right one to use for a given problem? Such a cheat sheet would help practitioners to be more strategic in expending resources to make forecasts and eventually improve the decisions that follow.
Why do these two categories matter?
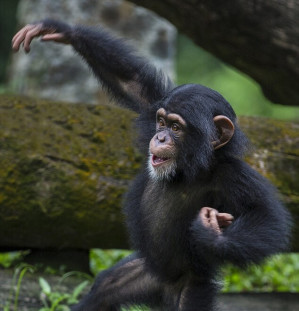
The first category — event characteristics — matters because if an event is incredibly difficult to forecast, then the forecasting method is irrelevant — success or failure becomes just a matter of chance. The behavior of the financial market, for example, is only predictable up to a point, and beyond that point there’s no real way of foreseeing the direction things will take. In this example, once we get beyond the limit of predictability, it barely matters whether the forecaster is a technical analyst, a fundamental analyst, or a dart-throwing chimp.
The second category — forecasting method — matters because when we employ an inappropriate method, events that are inherently predictable can erroneously look random. For example, doctors used to not know that dirty hands touching wounds could lead to fatal infections. Incidents of fatal infections seemed more unpredictable than they actually were because the “forecasting method” (i.e., medical knowledge) that doctors employed at the time was ignorant of the causal circuitry that underlies fatal infections. It’s interesting and useful to dissect the ways in which forecasting methods might be imperfect and to understand how they create distinct biases in the results. Flaws in forecasting methods are not equal.
Who might find your research interesting and why?
Practitioners who might find this research particularly interesting are those who operate in novel environments, such as R&D managers, entrepreneurs, early-stage investors, emerging market pioneers, and so on. One key idea that my research proposes is that it might be possible to foresee the difficulty of a forecasting task (again, to predict predictability) and also to pick the appropriate forecasting strategy without having to rely on comparables or historical data. Rather, forecasters might be able to rely on just the present attributes of the forecasting problem at hand.
This concept should sound appealing to, say, early-stage investors who are looking at a completely new market. While many investors can typically make forecasts about investment returns based on comparable investment types and historical performance data, early-stage investors in a completely new market often don’t have that luxury. What if an investment opportunity is not really comparable to anything else? What if there’s no relevant historical data for it?
My research suggests that we can get some sense about the difficulty of a forecasting task and also about the “smart” forecasting strategy to employ just by looking carefully at the existing attributes of the event in question. It’s an uplifting idea that appropriately treats forecasting as a present- and forward-looking endeavor, not a backward-looking one.
How did you conduct your research?
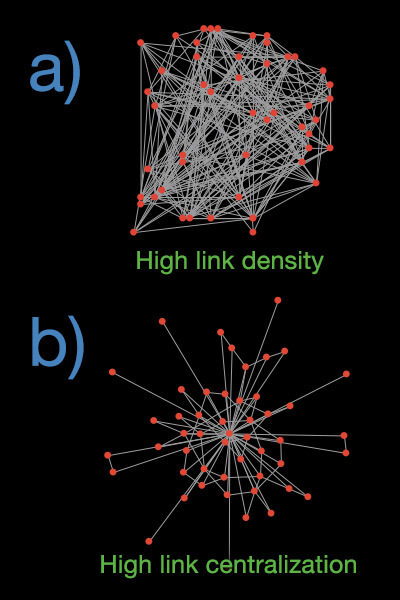
Method-wise, I use computational modeling and apply network theory to simulate different types of events as well as different aspects of forecasting methods that are commonly used to make forecasts about those events.
I base my investigative approach on one central idea: that each event has its own “structural signature.” Every event is preceded by another event; that preceding event is also preceded by another event, and so on. The preceding events are in other words precursors to the focal event. A focal event and its precursors are therefore linked by causal relationships, and these links/causal relationships can be readily depicted as a network diagram.
I focus on two structural properties of these networks: link density and level of centralization. If most of the precursors to a focal event are interdependent, they form a network with high link density (picture a dense hairball). And if most links in a network emanate from a handful of precursors, that network can be said to have a high level of centralization (picture a network that resembles a star, with only a few hubs from which most links emanate).
Tell us more about this “smart” forecasting strategy.
What I mean by that is that it might be possible indeed to make a reliable forecasting cheat sheet or a flowchart to show which event characteristics a forecaster should pay attention to first, second, third, and so on, in a way that is optimized for efficiency.
How would a forecaster know how to interpret the cheat sheet?
The key requirement is for the forecaster to have some understanding about two things: First, what are some precursors to the event in question? And second, how are those precursors causally related to one another?
While this sounds like a daunting requirement, my results actually show that a forecaster needs only to have some general idea about the overall structure of the causal relationships between the event precursors (i.e., how dense and how centralized the overall network of causal relationships is), not the exact relationships themselves. So, this is actually encouraging—it means that it might be possible to predict the predictability of an event without having complete knowledge about that event (which is virtually impossible in the real world), as long as the forecaster pays attention to a few of the right things.
And even though this sounds a bit surprising, it is actually consistent with what researchers have previously found, i.e., that simple heuristics are often enough for people to perform well in prediction tasks.
What kind of forecasting imperfections can this research can help us understand?
There are five different sources of forecasting imperfections that I look at in my study, all of which are common realities for forecasters:
- Ignorance about the causal structure of the event of interest, which is a very realistic source of imperfection. Rarely, if ever, do we know exactly how things are causally interrelated.
- Not having the luxury of being able to wait for more information to become available before making a forecast
- Not being able to attend to (and therefore utilize) all available information
- The tendency to clump pieces of information together into a big picture view, as opposed to treating each piece of information as distinct
- Attentional bias towards newer information, or the opposite, towards older information
My results offer some insights into when it’s relatively safe to, for example, be more ignorant about the causal structure of the event of interest, when it’s worth delaying making a forecast in order to wait for more information to become available, when it’s okay to look at the big picture, and so on.
It’s quite impossible to summarize all of these insights into just a few sentences, but the main point here is that if we know how the characteristics of forecasting methods interact with the characteristics of the event that is being forecasted, it’s possible to map out a “smart” forecasting strategy.
What do you see as the next steps?
Since my work is theoretical, the most important next step would be to validate it empirically. The key challenge is of course finding a research setting in which a network of causal relationships can be observed. Decision-making relationships among actors in a given organization, for instance, could be reasonable proxies for event causal relationships. Understanding the structural properties of the network that results from those decision-making relationships and looking at how they correspond to the predictability of the organization’s action would be a good start in empirical validation.
About the researcher
Wendy Ham received her PhD in 2014 from The Wharton School, University of Pennsylvania. In her dissertation, she looked at the ways in which computational models could be used to study information processing in organizational learning and innovation contexts, particularly those that are difficult to investigate empirically. While at Wharton, Wendy also worked with Prof. Martine Haas to study how seemingly irrelevant knowledge may be an important ingredient for breakthrough innovation. Wendy currently runs a technology and analytics development company in New York City. She can be reached at wendy.ham.wp14@wharton.upenn.edu.
The above research was done as part of her PhD dissertation, “Computational Models of Information Processing,” under the advice of Phil Tetlock and Dan Levinthal.