In the past decade, machine learning has become crucial in performing complex tasks such as big data analysis that we cannot do ourselves. In this episode of Mastering Innovation on SiriusXM Channel 132, Business Radio Powered by The Wharton School, Angela Zutavern, Managing Director at AlixPartners and author of The Mathematical Corporation, examines the extraordinary capabilities of machine learning when used in conjunction with our own minds.
Zutavern points out that even as artificial intelligence rapidly advances, there are still many categories where humans will continue outperforming machines for the foreseeable future. She discusses how machine learning can complement, not replace, humans’ creativity and problem-solving skills when applied to innovation efforts. Citing examples from Merck, the vaccine manufacturer, the International Hotels Group, and Microsoft, Zutavern emphasizes the possibilities for efficiency gains when companies implement artificial intelligence and machine learning effectively.
An excerpt of the interview is transcribed below. Listen to more episodes here.
Transcript
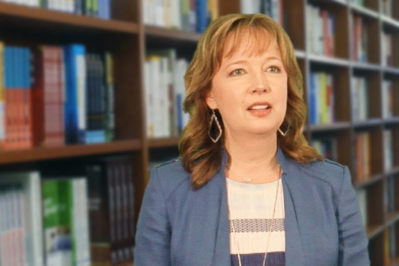
Nicolaj Siggelkow: Whenever we have new technologies, there is quite a lot of hype, a lot of buzzwords flying around, so let’s start out with some basics. Maybe you can help us sort of sift through some of these terms. People talk about machine intelligence and deep learning, but what does that mean? Can you help us all make a little more sense of these terms?
Angela Zutavern: Sure. For the past 50 years, we’ve been explicitly programming computers to carry out instructions. The big change now is that computers can learn on their own. This isn’t new; research has been going on for many, many years. What is new now is we have the computing power and data necessary to make artificial intelligence work.
I use artificial intelligence and machine intelligence as an umbrella term, meaning the concept of machines being able to take data and learn on their own from it. Then within that, there are specific types of algorithms and techniques, the broad category being machine learning, and within that you have deep learning and other areas.
Siggelkow: Let’s explore what are computers already good at. Where are the machines really intelligent?
Zutavern: Machines are outperforming people at many types of tasks. The main areas are anything having to do with comprehending a large set of data, perceiving detail, identifying patterns, any type of number crunching, remembering tasks, and documenting and organizing. That’s where it makes sense to really implement some of these types of technologies to free up people’s time to focus on the more innovative tasks. The areas where people are clearly outperforming machines include creativity, imagination, reasoning, problem-solving, and strategy.
“It makes sense to really implement some of these types of technologies to free up people’s time to focus on the more innovative tasks.” – Angela Zutavern
Siggelkow: Your belief is that the machines will not outperform on those dimensions too soon?
Zutavern: Machines learn from what happened in the past, so this is especially relevant to the innovation topic. Many people have asked me, “Why were none of the predictive models for the World Cup…you know, why were they so off?” None of the models were right; none of them were even close. It’s because some outcomes happened that had never happened in the past before. Same thing with innovation: you’re doing something for the first time ever. If there’s no past data to learn from, the artificial intelligence or the machine learning models won’t grasp those concepts.
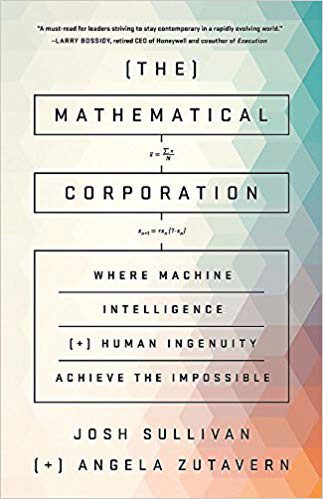
Siggelkow: The interesting thing is you started out with saying what computers are good at is data processing and seeing some patterns. That is sort of feeding into a decision-making process inside firms, right? That’s where it becomes interesting.
Computers are good at assessing data, but eventually, someone must make a decision, right? Of course, every firm would love to have a data-driven decision-making process. Everyone claims they have one. How do these firms move from data gathering and data analysis to insight, or at least impact decision-making? What’s the process like there?
Zutavern: I’ll give you an example of a project that Merck worked on. They’re one of the leading makers of human vaccines. They had a vaccine manufacturing process with four stages: growing yeast, agitating, fermenting, and purifying. Their problem wasn’t data; in fact, they had a ton of sensor data on all their manufacturing equipment.
What was happening in their manufacturing process was batches were getting ruined, and if something went wrong somewhere along the way, they had to throw out the entire batch. It’s very expensive when something goes wrong. They had data within each of those four phases, and what they hadn’t been able to do previously was combine the data across all four phases. This was due to the complexity; the computer power hadn’t been previously available.
When they combined the data across, it gave them completely new insight into what was causing the batches to go bad, and the results were unexpected. They originally suspected culprits like raw materials, but turned out to be some problems in the fermentation process. The complexity was such that, even with years of experience in this manufacturing environment, a person couldn’t look at all that data and come out with a conclusion, and the machine learning models were able to point in the right direction.
The real power, though, is from the combination of people and machines. The machine intelligence and the human intelligence together, that bring the best measurable business outcomes.
Siggelkow: It’s interesting. You said they already had the data, but they were just unable to see the pattern in the data, right?
Zutavern: They had 10 years’ worth of datasets from thousands of sensors — an amazing treasure trove of data. But until recently, we didn’t have the computer power, the algorithms, or the experience with machine learning that companies do today. They were lucky in that they had the raw dataset to learn from. Normally, you’re lucky if you get a couple years’ worth of data to build a model on, but to have 10 years’ worth of data was really impactful.
“One of the biggest mistakes that companies make is starting with a specific technology or specific data in mind.” – Angela Zutavern
Siggelkow: With the decline in cost of sensors, the cost of getting data is declining. Every firm is tempted to say, “Well, I heard data is important. Let’s collect more data,” and then the question is what to do with it. How do you even think about a strategy of collecting data? I see some firms say, “Well, let’s just collect everything that we can.” Then other firms say, “Well, I have a precise model in my mind of what the relevant data is and I only want to capture that,” so that probably is also not the right approach, right? You want to let the machines see patterns that you didn’t know about, but at the same time, you don’t want to drown in data?
Zutavern: One of the biggest mistakes that companies make is starting with a specific technology or specific data in mind. Instead, successful approaches start with the business problem. What problem are you trying to solve? Save money, increase revenue, whatever the case may be. That business problem has got to drive the approach both for the technology that you implement and the data that you collect.
About Our Guest
Angela pioneered the application of machine intelligence to business leadership and strategy. She is a highly regarded leader with more than 25 years of experience in artificial intelligence (AI) and other areas of digital consulting, including machine learning, data solutions, and related tools. Angela works with clients to help them implement robust and sophisticated digital solutions that drive effective, data-led decision making. She specializes in technology-based transformations, including cost reduction and efficiency efforts as well as capability-building and innovation-based growth plays. Angela is a frequent speaker on the power of AI and coauthor of “The Mathematical Corporation: Where Machine Intelligence and Human Ingenuity Achieve the Impossible,” an 800-CEO-Read Business Book Awards recommendation.
Mastering Innovation is live on Thursdays at 4:00 p.m. ET. Listen to more episodes here.