Artificial Intelligence has emerged as one of the most transformative technologies of our time, reshaping industries and redefining the competitive landscape. Yet the complexities and costs associated with AI development and deployment, coupled with the uncertainty of value creation, make it clear that no organization can fully realize AI’s potential in isolation. This reality has spurred the formation of innovation ecosystems in which diverse organizations collaborate to harness complementarities, share resources, and drive collective progress. Moreover, like any general-purpose technology, AI disrupts existing innovation ecosystems such as robotics, new drug development, and bioengineering.
Against this backdrop, the Mack Institute brought leading Wharton researchers and business practitioners to our Philadelphia campus for our Fall 2024 Conference: AI-Driven Innovation Ecosystems. The following report sums up key takeaways from the day-long event.
Understanding Wharton’s Ecosystem Framework
The conference opened with a presentation by Prof. John Paul MacDuffie (Wharton), who introduced the research underpinning ecosystem theory. While the term “ecosystem” is commonly used as a metaphor in business and social sciences, Wharton scholars have developed it into a structured framework to analyze the collaborative networks that drive innovation and value creation. This framework provides a systematic way to examine modular architectures, value propositions, bottlenecks, and interdependencies and complementarities that characterize innovation ecosystems.
Note: Prof. MacDuffie’s presentation was based in part on the research of Wharton’s Prof. Rahul Kapoor. Please see his papers (1, 2) for more details.
Defining an Ecosystem
A business ecosystem is a group of different actors (such as firms, individuals, or other organizations) that collaborate to create and deliver value for a specific product or service. Ecosystems are further characterized by:
- A lack of strict hierarchy (no one actor has total control).
- Dependence on complementarities (actors work together to enhance the overall value)
- Structural interdependencies between actors (connections that are technological, organizational, or both)
Crucially, an ecosystem is not the same as a supply chain or value chain. Supply chains are typically hierarchical, and value chains are confined to a single firm.
MacDuffie illustrated this distinction using Apple’s iPhone as an example. Apple’s supply chain comprises its global network of hardware and assembly suppliers, designed to meet demand efficiently and keep costs low. Its value chain is the investments it makes internally in R&D, marketing, and distribution. The ecosystem (of which iPhone is the “core product” or “focal offering’) is the network of app developers, accessory manufacturers and other service providers that increase the overall value proposition of the iPhone.
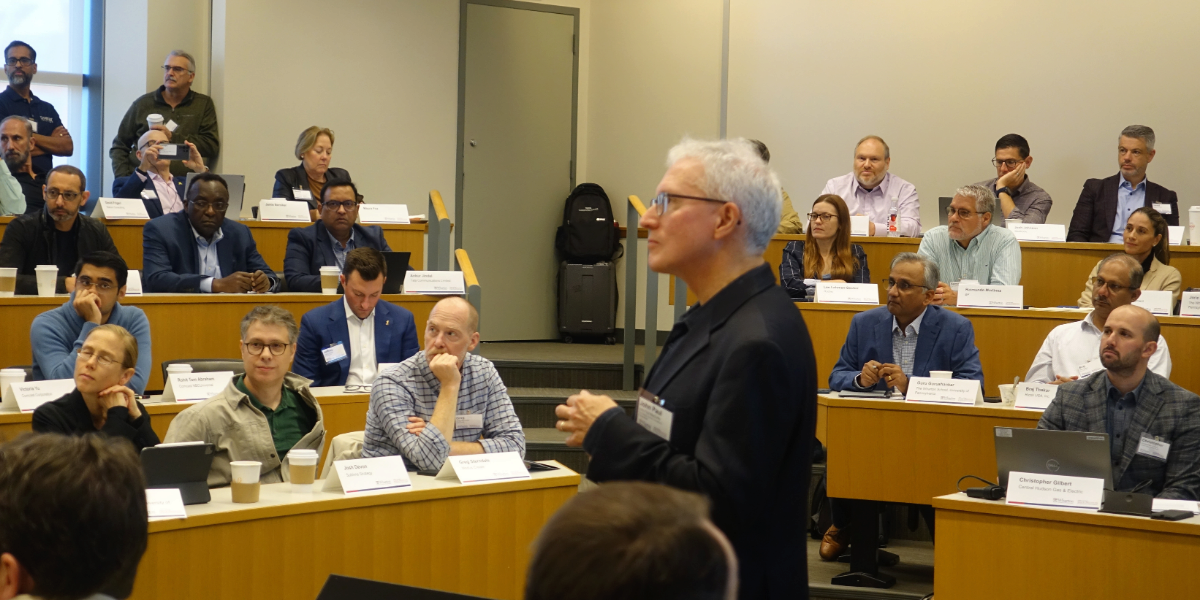
Activities, Actors, Architectures
This framework breaks down ecosystems into three core components: activities (what needs to happen), actors (who is involved) and architectures (how they are connected). MacDuffie explained these “Three A’s” using Tesla as an example:
Activities are the actions or processes necessary to deliver the ecosystem’s value proposition, such as production, distribution, and support. In Tesla’s ecosystem, activities include designing batteries, manufacturing vehicles, building charging stations, and developing software.
Actors are the participants and stakeholders in a given system. They include the focal firm (Tesla, in this example), complementors (partners who add value to the focal firm, such as Panasonic, Tesla’s battery partner) and orchestrators (entities that coordinate the system).
Architectures are the structural and organizational design of the ecosystem. This includes technological architecture as well as organizational architecture (e.g. partnerships, contracts, governance models). Architectures can range from highly interdependent (integral) designs, in which components are tightly interconnected, to more modular structures, in which components operate independently.
Bottlenecks
MacDuffie emphasized the importance of identifying and addressing bottlenecks to build effective ecosystem partnerships. For example, Tesla identified batteries as a key bottleneck in electric vehicle adoption. By partnering with Panasonic, who had previous experience creating batteries for Toyota’s Prius, they were able to successfully scale up production. In this ecosystem, Tesla is the focal firm and Panasonic is the complementor. They both benefit from this partnership: Tesla from increased production and Panasonic from capturing value in the emerging EV market.
Thus, bottlenecks are opportunities for complementary value creation that drive the evolution of any innovation ecosystem.
Insights From Industry Panels
OpenAI and Amazon Web Services: How Do Core Technology Providers Contribute to Ecosystems?
Amazon Web Services (AWS) and OpenAI are essential digital infrastructure providers for thousands of companies worldwide, from global giants like Microsoft and Netflix to emerging startups. This unique position means they serve a dual role: each acts as a complementor, supporting a wide range of ecosystems, while simultaneously serving as an orchestrator, shaping an ecosystem around their own offerings.
Panelists Juston Forte (Lead Solutions Architect, OpenAI) and Patrick Combes (Senior Principal of Technology, Amazon) began the discussion by explaining how their respective companies define the core value proposition within their own ecosystems. Combes described AWS as a utility provider, emphasizing its ability to deliver scalable, modular, and customizable solutions that adapt to clients’ specific needs. Bedrock, AWS’ Gen AI-based toolkit, was designed with this model in mind.
“When generative AI technologies began to roll out, we had to reassess how to build a service that aligned with our core principles of price, selection, and convenience,” explained Combes. “That’s why Bedrock doesn’t offer access to just one model or a single solution; instead, it provides access to multiple models, all through a unified API. This ensures customers can easily access and work with the models they need, enhancing convenience and offering a broad selection at the lowest possible cost.”
AWS’s AI offerings expand the capabilities of its existing cloud infrastructure, so customers can build and customize their own innovative products and services. For example, Amazon’s partnership with NVIDIA allows them to integrate their hardware into the AWS platform.
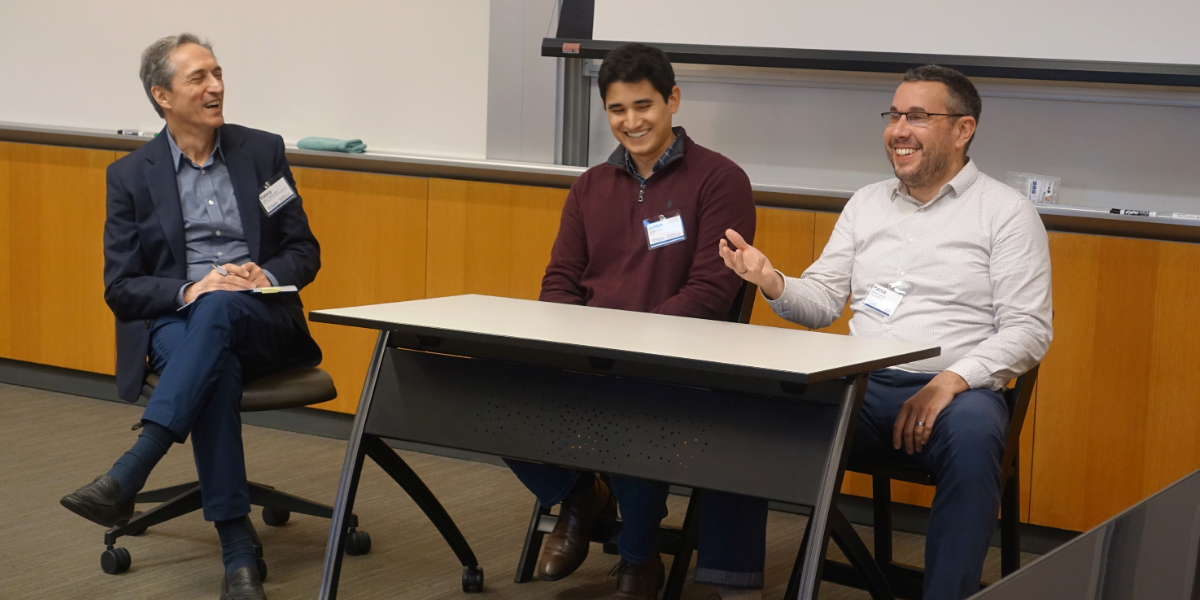
In contrast, Forte explained that OpenAI positions itself as an AI platform provider, with its proprietary large language models (LLMs) powered by cutting-edge research. While OpenAI’s tools enable many businesses to build innovative applications, he cautioned that businesses should continue to invest in their core product features to capture the full value of AI models.
“When you approach building with our models, you shouldn’t think about the models themselves as your moat, but instead the other aspects that you build around it,” said Forte. “You have to go back to the basic elements of a successful SaaS offering. What is the user interface like? What specific expertise are you bringing to the table? This will help you differentiate from the next startup building with these same intelligence models.”
Forte stressed that this will only become more important as AI technology itself becomes more ubiquitous. Eventually, the products we interact with every day will all utilize AI technology and having those features will not be a differentiator, but a basic expectation.
“Having intelligence built-in will not be your moat,” he said. “It will be an expectation at a certain point. Even now, more people are getting used to ‘the ChatGPT experience’ and leaving platforms that don’t offer it.”
Combes and Forte also spoke extensively about AI accountability, with one attendee suggesting that it should be the “Fourth A” alongside actor, activity and architecture. Combes suggested that a flexible “shared responsibility” model can help firms meet changing security needs and regulations.
“In the context of generative AI, this means providing tools and mechanisms that enable customers to meet their own governance and regulatory needs,” he said. “For example, with AWS Bedrock, we’ve built guardrails to limit model outputs and ensure they comply with specific customer requirements. These guardrails can be tailored to a variety of frameworks, allowing customers to align with evolving standards and regulations without needing to redesign their entire system.”
While OpenAI builds accountability features into the model itself (such as automatic refusal of dangerous requests), Forte says that the broader community plays an important role in helping “stress test” ChatGPT to ensure it is as safe as possible. This can include feedback from security professionals like red teamers and enterprises that use ChatGPT.
“When we work with enterprises, we guide them into building guardrails according to their specific industry regulations,” said Forte. “This strengthens our security and accountability initiative overall. Once the model is being used in different contexts, by different kinds of people, we’re able to get better feedback on where the safety gaps are.” In other words, ecosystems themselves can help improve safety and accountability through their very structure.
Combes summarized the overall value of ecosystems as helping firms excel where they might otherwise face limitations or struggle to operate. He says this value is particularly important for core technology providers, because the sheer size of firms like Amazon can itself be a bottleneck.
“To put it plainly, we’re a very large organization,” he said. “Our size makes it difficult to operate effectively in niche or vertical spaces—those aren’t necessarily our areas of expertise or primary strengths. Instead, we rely on our relationships with providers and partners to fill those gaps. They focus on the specific areas where we know we can’t operate as quickly as our customers may need. Our partners and customers are key to bringing focus and specialization to these areas.”
Automation Anywhere: Creating Value with AI Agents
As artificial intelligence continues to advance, AI agents—systems or programs capable of making decisions and performing actions with minimal human involvement—are poised for widespread adoption. Their ability to automate complex, repetitive, and decision-driven tasks positions them as transformative tools for reshaping organizational operations.
Tejasvi Devaru (Automation Anywhere) joined Prof. David Hsu (Wharton) for a fireside chat to discuss the potential impact of emerging AI agents. Devaru, who is actively working on implementing AI agents as his company’s innovative product, highlighted their potential to significantly boost productivity, particularly in daily office workflows.
“One of the use cases that we are trying internally is for sales representative,” Devaru explained. “A sales rep’s typical day is probably first checking email and taking action based on what’s in his inbox. This could include updating contact information, responding to new leads by creating or updating opportunities, or scheduling meetings. The sales rep may also research market trends, track major events affecting their customers, and prepare for outreach. Finally, their day probably involves negotiation and closing deals.”
An AI agent trained on these “microtasks” could not only assist with this work but take over much of it, enhancing productivity across the business. Devaru elaborated on this vision:
“The future vision for me is this: a sales rep comes into the office, opens their laptop, and an AI agent presents a summary of the day,” he said. “For example: ‘You have 20 emails—10 are spam and have been filtered out. Two emails required updates to contact information, and those updates have already been made. Follow-up actions have been taken for three others, and meetings have been scheduled. Here are two remaining items that need your attention.’”
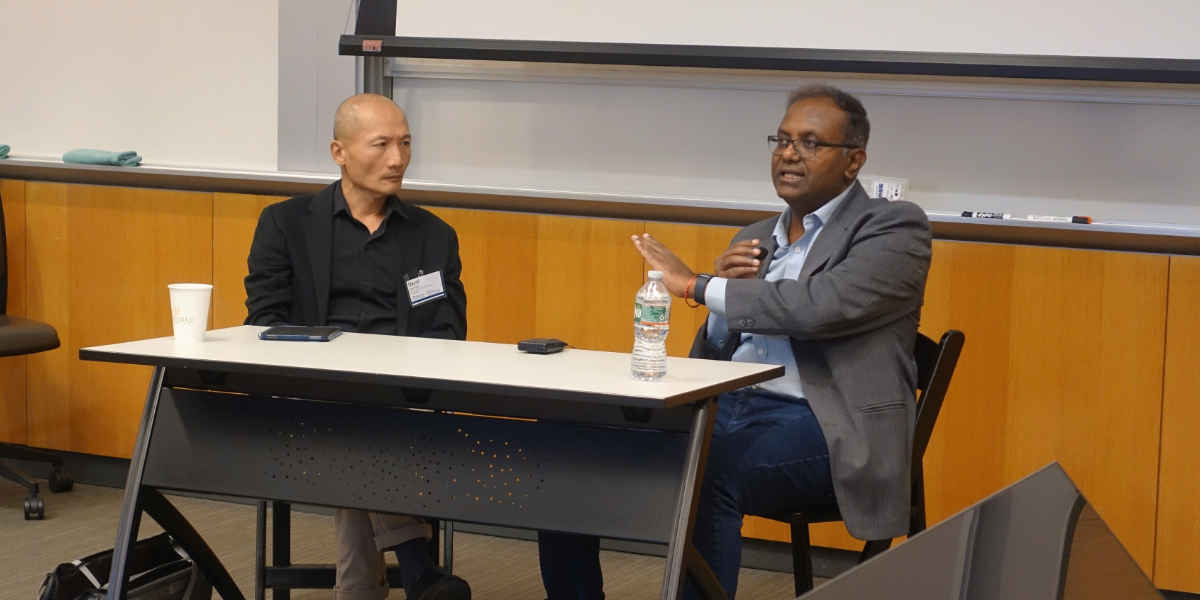
As AI technology continues to develop, the AI agent ecosystem evolves and changes. Hsu and Devaru discussed what form the emerging ecosystem will take. Will it remain a unified, monolithic system, or will it segment by industry or function? Should development prioritize general-purpose tools that cater to a wide range of applications, or focus on specialized, application-specific solutions?
Devaru believes orchestrators are already emerging, but he is certain the ecosystem will not be highly centralized and hierarchical, as it will require flexibility to accommodate diverse tools, applications, and stakeholders.
“The ecosystem will not be monolithic; instead, it requires a service-oriented or, ideally, a microservices architecture,” he said. “For example, if an AI agent needs to execute several tasks, you want the LLM to think independently about each one and execute them separately. Bundling them into a single operation would limit flexibility. Microservices architecture allows the AI to handle diverse scenarios more effectively.”
Yet the need for bespoke microservices complicates the development of a unified business model. Hsu raised the possibility of creating an off-the-shelf system for AI agents, akin to Apple’s app store. Devaru noted that, while businesses often use automation for common tasks (e.g., invoice generation), each business has its own nuances that make a “plug and play” model difficult to implement.
“Right now, we’re in the middle of the spectrum between manual workflows and complete AI autonomy,” said Devaru. “Eventually, we will get to 80-90% automation, unleashing more productive hours for employees to do what humans are meant to do: more strategic thinking, relationship management.”
Insights From Small Group Roundtables
Half of our conference was dedicated to group discussions, in which participants gathered by industry or vertical to discuss how to turn ideas from the opening sessions into actionable takeaways. After a few hours of in-depth deliberations, all the participants gathered for the groups’ summary presentations led by Prof. Rahul Kapoor, one of the authors of Wharton’s innovation ecosystems framework. Below are key insights across each of the four groups: LLM and Clouds, Life Sciences & Healthcare, AI Agents and Ecosystems, and Autonomous and Connected Vehicles.
Trust and Collaboration are Foundational Challenges Across Ecosystems
Across all the groups, trust was named as the most critical factor. It affects data sharing, collaboration, adoption of innovative technologies and, ultimately, complementary value creation and ecosystem emergence. Trust issues stem from unclear data ownership, legal uncertainties, and concerns over intellectual property.
To be an effective ecosystem participant, organizations must navigate the delicate balance between sharing data, safeguarding competitive advantages, and fostering collaboration. A lack of ecosystem competence—understanding what to share, what to protect, and how to collaborate effectively—undermines trust and hinders progress.
Breaking Data Silos and Encouraging Data Collaboration
Too often, data is siloed across institutions and stakeholders, particularly in life sciences and healthcare. Breaking down these silos is essential to unlocking the full potential of AI and fostering collaboration. Incentives for sharing data and strong governance frameworks are critical.
Furthermore, a fair mechanism for sharing the value generated from shared data—whether through revenue splits or informed consent—can incentivize stakeholders to collaborate. This approach enables more robust AI models, better automation, and greater overall ecosystem value.
Regulations and Standards Must Balance Innovation and Safety
Government regulations are both a constraint and an enabler. Standardized frameworks can establish trust and accelerate adoption while preventing monopolistic practices, particularly in sectors where people are concerned about safety, such as the automotive industry. However, overly strict or fragmented regulatory environments can slow progress.
Likewise, industry standards—like those established in telecom and aerospace—help guide R&D, facilitate market entry, and ensure interoperability. However, overly large or competitive standard-setting bodies can stall progress by creating inefficiencies or conflicts among participants.
Geopolitics and Local Contexts Impact Ecosystem Evolution
Geopolitical factors and regional variations—such as infrastructure readiness, consumer attitudes, and legal regimes—shape the evolution of ecosystems. Countries with fewer legacy systems, like China, have an advantage in leapfrogging to advanced technologies, while developed nations face more incremental progress.
Wrapping Up
Dr. Valery Yakubovich, Executive Director of the Mack Institute, summarized the conference in terms of Mack Institute’s mission: ”We support Wharton faculty’s research and translate it into experiential learning and real-world business practice. At this conference, participants engaged directly with the authors of Wharton’s innovation ecosystem framework, learning firsthand insights to apply in their own practice. The key takeaway is that the future of AI-driven ecosystems lies in leveraging complementarities while fostering trust and transparency, breaking down silos, and creating value for all ecosystem members. Understand your role within the ecosystem and be a good steward of it—then, innovation will flourish.”
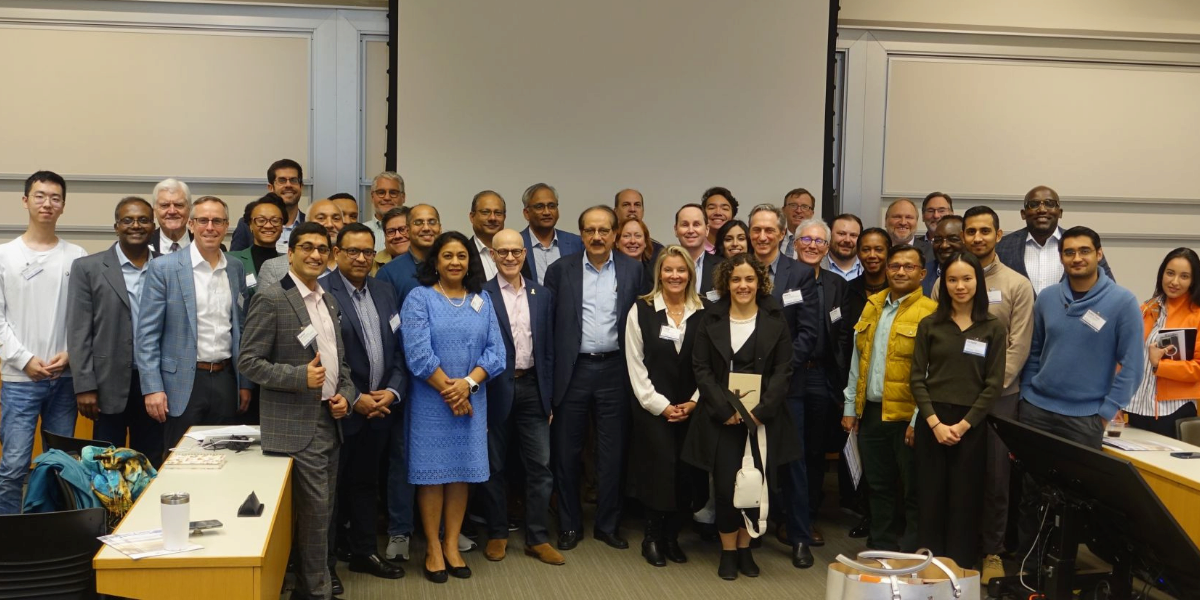